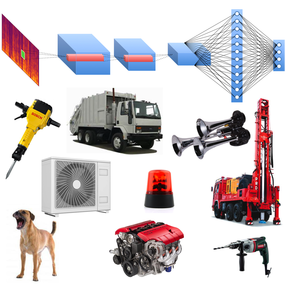
For further details see our paper:
Deep Convolutional Neural Networks and Data Augmentation For Environmental Sound Classification
J. Salamon and J. P. Bello
IEEE Signal Processing Letters, In Press, 2017.
[IEEE][PDF][BibTeX][Copyright]
Deep Convolutional Neural Networks and Data Augmentation For Environmental Sound Classification20/1/2017 ![]()
The ability of deep convolutional neural networks (CNN) to learn discriminative spectro-temporal patterns makes them well suited to environmental sound classification. However, the relative scarcity of labeled data has impeded the exploitation of this family of high-capacity models. This study has two primary contributions: first, we propose a deep convolutional neural network architecture for environmental sound classification. Second, we propose the use of audio data augmentation for overcoming the problem of data scarcity and explore the influence of different augmentations on the performance of the proposed CNN architecture. Combined with data augmentation, the proposed model produces state-of-the-art results for environmental sound classification. We show that the improved performance stems from the combination of a deep, high-capacity model and an augmented training set: this combination outperforms both the proposed CNN without augmentation and a “shallow” dictionary learning model with augmentation. Finally, we examine the influence of each augmentation on the model’s classification accuracy for each class, and observe that the accuracy for each class is influenced differently by each augmentation, suggesting that the performance of the model could be improved further by applying class-conditional data augmentation.
For further details see our paper: Deep Convolutional Neural Networks and Data Augmentation For Environmental Sound Classification J. Salamon and J. P. Bello IEEE Signal Processing Letters, In Press, 2017. [IEEE][PDF][BibTeX][Copyright]
0 Comments
![]()
Automated classification of organisms to species based on their vocalizations would contribute tremendously to abilities to monitor biodiversity, with a wide range of applications in the field of ecology. In particular, automated classification of migrating birds’ flight calls could yield new biological insights and conservation applications for birds that vocalize during migration. In this paper we explore state-of-the-art classification techniques for large-vocabulary bird species classification from flight calls. In particular, we contrast a “shallow learning” approach based on unsupervised dictionary learning with a deep convolutional neural network combined with data augmentation. We show that the two models perform comparably on a dataset of 5428 flight calls spanning 43 different species, with both significantly outperforming an MFCC baseline. Finally, we show that by combining the models using a simple late-fusion approach we can further improve the results, obtaining a state-of-the-art classification accuracy of 0.96.
Fusing Shallow and Deep Learning for Bioacoustic Bird Species Classification J. Salamon, J. P. Bello, A. Farnsworth and S. Kelling In IEEE International Conference on Acoustics, Speech and Signal Processing (ICASSP), New Orleans, USA, March 2017. [IEEE][PDF][BibTeX][Copyright]
CLO-43SD is targeted at the closed-set N-class problem (identify which of of these 43 known species produced the flight call in this clip), while CLO-WTSP and CLO-SWTH are targeted at the binary open-set problem (given a clip determine whether it contains a flight call from the target species or not). The latter two come pre-sorted into two subsets: Fall 2014 and Spring 2015. In our study we used the fall subset for training and the spring subset for testing, simulating adversarial yet realistic conditions that require a high level of model generalization. For further details about the datasets see our article: Towards the Automatic Classification of Avian Flight Calls for Bioacoustic Monitoring J. Salamon , J. P. Bello, A. Farnsworth, M. Robbins, S. Keen, H. Klinck and S. Kelling PLOS ONE 11(11): e0166866, 2016. doi: 10.1371/journal.pone.0166866. [PLOS ONE][PDF][BibTeX] You can download all 3 datasets from the Dryad Digital Repository at this link. ![]()
Automatic classification of animal vocalizations has great potential to enhance the monitoring of species movements and behaviors. This is particularly true for monitoring nocturnal bird migration, where automated classification of migrants’ flight calls could yield new biological insights and conservation applications for birds that vocalize during migration. In this paper we investigate the automatic classification of bird species from flight calls, and in particular the relationship between two different problem formulations commonly found in the literature: classifying a short clip containing one of a fixed set of known species (N-class problem) and the continuous monitoring problem, the latter of which is relevant to migration monitoring. We implemented a state-of-the-art audio classification model based on unsupervised feature learning and evaluated it on three novel datasets, one for studying the N-class problem including over 5000 flight calls from 43 different species, and two realistic datasets for studying the monitoring scenario comprising hundreds of thousands of audio clips that were compiled by means of remote acoustic sensors deployed in the field during two migration seasons. We show that the model achieves high accuracy when classifying a clip to one of N known species, even for a large number of species. In contrast, the model does not perform as well in the continuous monitoring case. Through a detailed error analysis (that included full expert review of false positives and negatives) we show the model is confounded by varying background noise conditions and previously unseen vocalizations. We also show that the model needs to be parameterized and benchmarked differently for the continuous monitoring scenario. Finally, we show that despite the reduced performance, given the right conditions the model can still characterize the migration pattern of a specific species. The paper concludes with directions for future research.
The full article is available freely (open access) on PLOS ONE: Towards the Automatic Classification of Avian Flight Calls for Bioacoustic Monitoring J. Salamon , J. P. Bello, A. Farnsworth, M. Robbins, S. Keen, H. Klinck and S. Kelling PLOS ONE 11(11): e0166866, 2016. doi: 10.1371/journal.pone.0166866. [PLOS ONE][PDF][BibTeX] Along with this study, we have also published the three new datasets for bioacoustic machine learning that were compiled for this study. ![]() Today SONYC was featured on several major news outlets including the New York Times, NPR and Wired! This follows NYU's press release about the official launch of the SONYC project. Needless to say I'm thrilled about the coverage the project's launch is receiving. Hopefully it is a sign of the great things yet to come from this project, though, I should note, it has already resulted in several scientific publications. Here's the complete list of media articles (that I could find) covering SONYC. The WNYC radio segment includes a few words from yours truly :) BBC World Service - World Update (first minute, then from 36:21) Sounds of New York City (German Public Radio) If you're interested to learn more about the SONYC project have a look at the SONYC website. You can also check out the SONYC intro video: I'm extremely excited to report that our Sounds of New York City (SONYC) project has been granted a Frontier award from the National Science Foundation (NSF) as part of its initiative to advance research in cyber-physical systems as detailed in the NSF’s press release. NYU has issued a press release providing further information about the SONYC project and the award. From the NYU press release: The project – which involves large-scale noise monitoring – leverages the latest in machine learning technology, big data analysis, and citizen science reporting to more effectively monitor, analyze, and mitigate urban noise pollution. Known as Sounds of New York City (SONYC), this multi-year project has received a $4.6 million grant from the National Science Foundation and has the support of City health and environmental agencies. Further information about the project project can be found on the SONYC website. You can also check out the SONYC intro video: ![]() Our BirdVox project has been awarded a $1.5 million Big Data program grant, awarded to the project BirdVox: Automatic Bird Species Identification from Flight Calls, conducted jointly by NYU and the Cornell Lab of Ornithology (CLO), who lead the project. Here's an excerpt from the NYU press release: Collecting reliable, real-time data on the migratory patterns of birds can help foster more effective conservation practices, and – when correlated with other data – provide insight into important environmental phenomena. Scientists at CLO currently rely on information from weather surveillance radar, as well as reporting data from over 400,000 active birdwatchers, one of the largest and longest-standing citizen science networks in existence. However, there are important gaps in this information since radar imaging cannot differentiate between species, and most birds migrate at night, unobserved by citizen scientists. The combination of acoustic sensing and machine listening in this project addresses these shortcomings, providing valuable species-specific data that can help biologists complete the bird migration puzzle. For further information about the project check out the BirdVox website.
Following BirdVox's appearance on the Science Friday radio show, Public Radio International (PRI) has published a follow-up article about BirdVox: "Scientists are using sound to track nighttime bird migration". Here's an excerpt: A group of researchers at New York University and the Cornell Lab of Ornithology are helping to track the nighttime migratory patterns of birds by teaching a computer to recognize their flight calls. The technique, called acoustic monitoring, has existed for some time, but the development of advanced computer algorithms may provide researchers with better information than they have gathered in the past. ![]() You can read the full article here, and listen to the Science Friday interview here. Further information about the project can be found on the BirdVox website. On Friday June 24th the popular Science Friday radio show featured a segment about our BirdVox project. The segment included sound bites from fellow BirdVox researcher Andrew Farnsworth and myself, followed by a live interview with Juan Pablo Bello.
In the segment we discuss our latest work on automatic bird species classification from flight call recordings captured with acoustic sensors for bioacoustics migration monitoring. You can listen to the BirdVox segment here. ![]() Today I'll be giving an invited talk at the Machine Learning for Music Discovery Workshop as part of the ICML 2016 conference. The talk is about Pitch Analysis for Active Music Discovery: A significant proportion of commercial music is comprised of pitched content: a melody, a bass line, a famous guitar solo, etc. Consequently, algorithms that are capable of extracting and understanding this type of pitched content open up numerous opportunities for active music discovery, ranging from query-by-humming to musical-feature-based exploration of Indian art music or recommendation based on singing style. In this talk I will describe some of my work on algorithms for pitch content analysis of music audio signals and their application to music discovery, the role of machine learning in these algorithms, and the challenge posed by the scarcity of labeled data and how we may address it. And here's the extended abstract: Pitch Analysis for Active Music Discovery J. Salamon Machine Learning for Music Discovery workshop, International Conference on Machine Learning (ICML), invited talk, New York City, NY, USA, June 2016. [PDF] The workshop has a great program lined up, if your'e attending ICML 2016 be sure to drop by! |
NEWSMachine listening research, code, data & hacks! Archives
March 2023
Categories
All
|